In today’s data-driven world, a robust data management strategy has become as crucial as a sound business strategy. For companies across all sectors of the economy, capitalising on data-driven insights is a defining characteristic of companies at the forefront of their markets. Nowhere is this more apparent than in the life sciences industry, where the accuracy and reproducibility of data can make a life-saving difference. The dependability of results can make or break a product, and effective use of data can save years of development and validation time, or determine the success of a study. Here we explore the significance of effective data management in life sciences and its impact on product development, testing, and the overall success of studies.
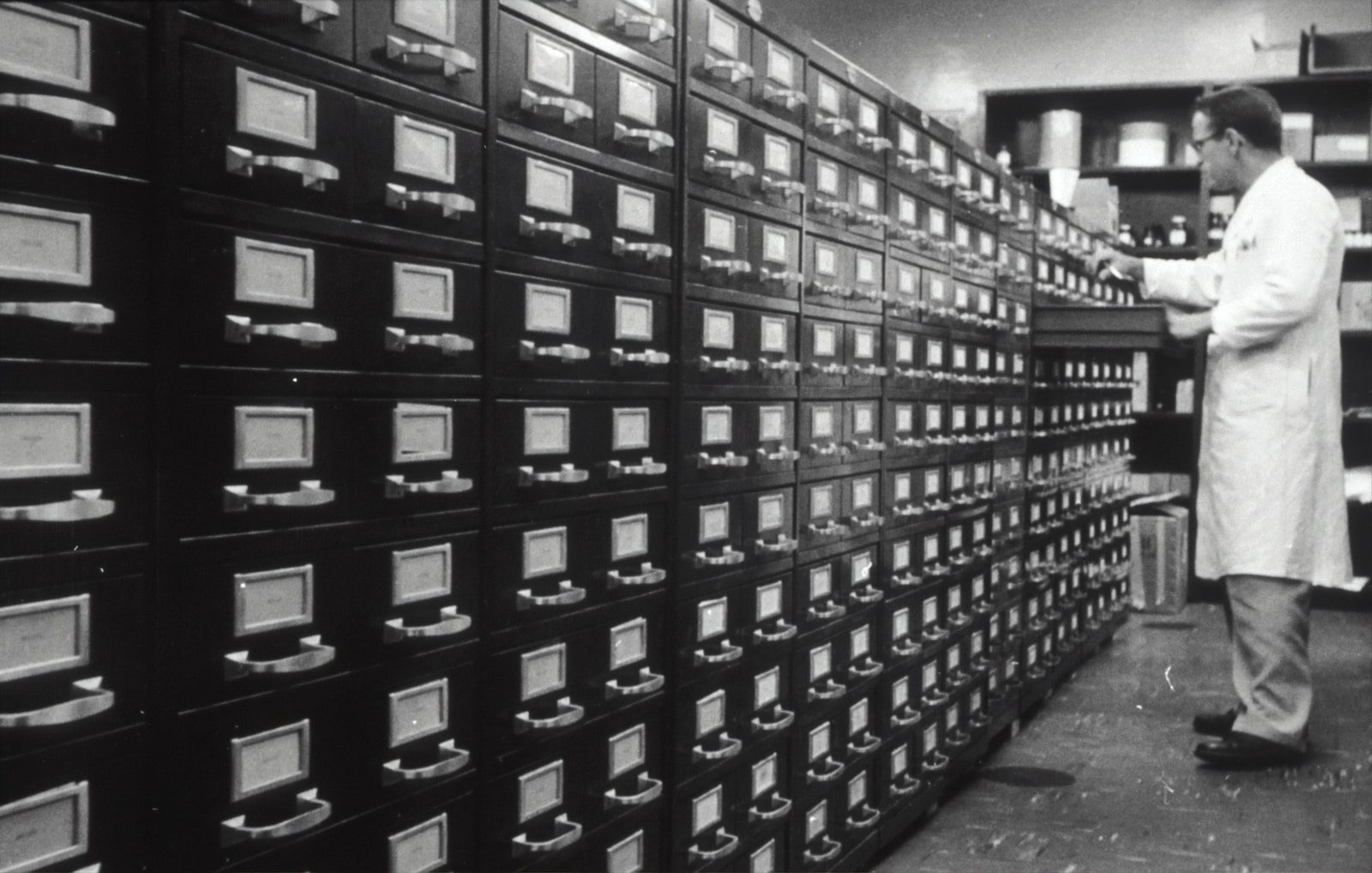
The Role of Data Management in Life Sciences:
Data management spans the workflow, from data acquisition to data processing and storage. Each step plays a crucial role in ensuring the reliability and usability of data for making informed decisions.
- Data Acquisition:
The first step in effective data management is to assess whether the collected data addresses the research question at hand. It is critical to gather the right data with relevant controls to minimize biases and confounding factors. This ensures that the data collected is fit for its purpose and can yield accurate insights. - Data Processing:
The life sciences generate vast amounts of data, making it essential to have a clear overview of the data’s contents and the ability to extract specific information efficiently. A data processing workflow to structure data free of duplications and with quality controls is essential to enable knowledge gain from collected data. - Data Storage and Update:
Data must be readily accessible and compatible with various AI algorithms and standard protocols. Additionally, processing workflows and the storage structure should be flexible enough to incorporate new results rapidly and address new and evolving questions as goals change over time. This adaptability ensures that data remains relevant and valuable for years to come.
Effective data management is best achieved by integrating domain expertise in the field of study with the appropriate data handling approaches to ensure data is relevant, accessible, and usable.
Maximising the Value of Your Data:
To fully leverage the potential of data in the life sciences, organisations must prioritise effective data management:
- Integrate Domain Expertise and Data Handling:
The ideal approach is to merge domain expertise in the field of study with appropriate data handling methods. This combination ensures that the data collected is relevant, accessible, and usable, leading to meaningful insights and informed decision-making. - Embrace Usability and Future Readiness.
Data usability is key to deriving value from the collected information. Focusing on data organisation and developing standardised workflows to unlock the full potential of data. Moreover, preparing data for future applications guarantees that it remains valuable even as objectives evolve.
Conclusion:
In the rapidly evolving landscape of life sciences, an effective data management strategy is not just a nice-to-have but a necessity for success. Whether it’s driving product development, ensuring reproducibility in studies, or saving time and resources, data management can make a significant difference. By investing in data acquisition, processing, and storage, companies can position themselves at the forefront of their market and make meaningful contributions to the advancement of science and healthcare.