In the realm of medicine, the pursuit of “truth” is a multifaceted challenge shaped by varying perspectives and biological complexities. Defining the “right answer” in medicine is therefore not a straightforward task, as it encompasses a dynamic interplay of factors that don’t always conform to conventional metrics and standard definitions. Furthermore, you can only attempt to obtain the “right answer” if you ask the “right question” in the first place.
Unlike in some fields, there’s often not a definitive “ground truth” for a medical entity. Biology constitutes a spectrum, and while statistical averages and metrics provide a general framework, they can fail to capture the true diversity of patients. The variability of human physiology, the diversity of individual responses to treatments, and the nuanced nature of illnesses mean that no one is truly “average”. “Average” is simply a reference born from statistical convenience, that in fact represents no single individual. Similarly, outliers are “truthful” cases. Hence an analysis overly focused on average metrics can conceal important clues lying in the variability.
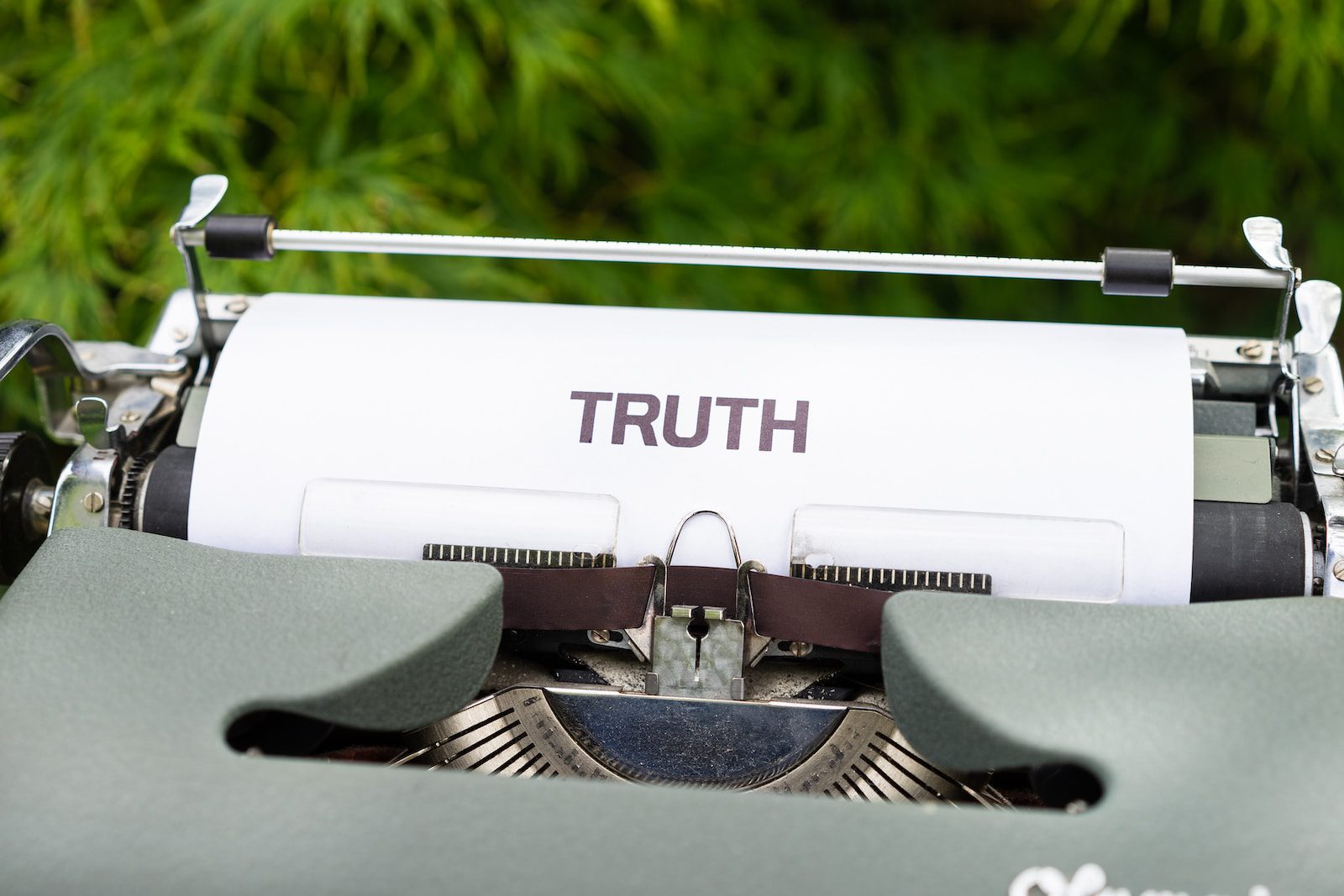
The notion of “truth” further fragments when considering the perspective of different experts. Each medical professional bases decisions upon their own experiences, biases, and viewpoints. Consequently, the interpretation of what constitutes the “true” label may vary among experts. Thus, the question should be posed as how likely something is to be true, rather than simply is it true or not.
In the pursuit of the “right answer”, it is especially prudent in medicine to not lose sight of the inherent diversity of biology and remember that average metrics and reference ranges are born from mathematical convention and are not synonymous with “ground truths”.
Data quality plays a key role in shaping the “truth” in medicine. Biases, errors, and incomplete information can skew average or median metrics as well as interpretation of individual cases. Therefore, data quality control and informed consideration of bias are crucial to mitigate against errors in interpretation and extract meaningful insights.