Addressing the pressing challenges underscored at the Intelligent Health AI conference, the foremost hurdle in ushering in big data- and AI-driven drug discovery and healthcare delivery lies in bridging the chasm between digitised health data and its effective utilisation.
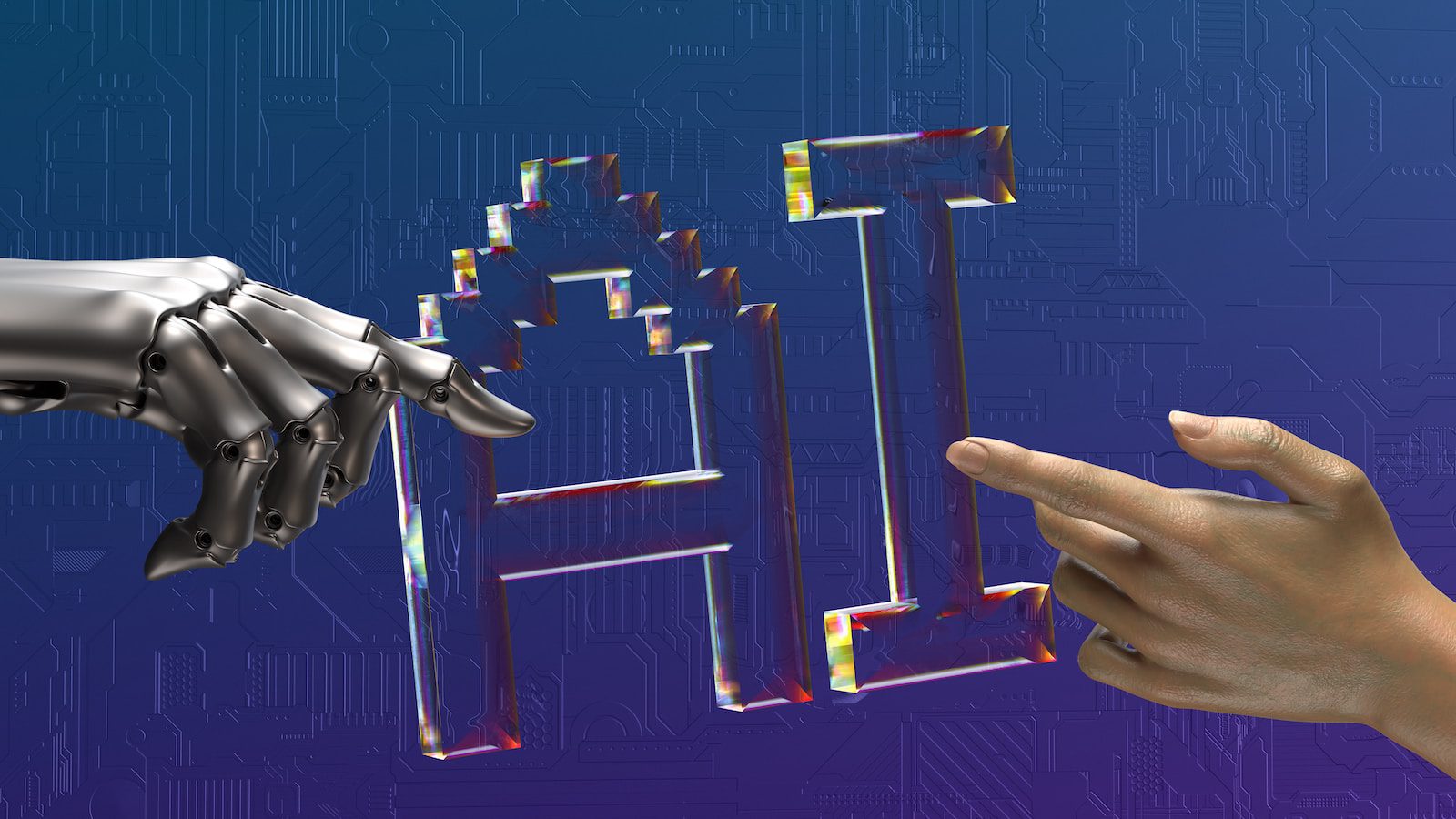
This conundrum becomes especially pronounced when dealing with the vast reservoirs of real-world medical data collected daily across global health centers. This data trove is riddled with randomly scattered errors, non-systematic inconsistencies, and even self-contradicting entries, rendering the notion of “automatic cleaning” impractical, with the potential to exacerbate errors.
Automation can shoulder a substantial burden in data extraction and standardisation, yet it pales in comparison to the robustness achieved through targeted error correction workflows informed by domain expertise. The linchpin here is the incorporation of human oversight quality controls into the data curation process, an indispensable element of data- and AI-driven solutions. Such an approach not only assures data quality and integrity but also fosters reproducibility and engenders trust.
In essence, the foremost challenge of harnessing data for transformative health advancement lies in navigating the intricate terrain between raw digitised data and its effective utilisation, a journey that demands a judicious fusion of automation and human expertise.